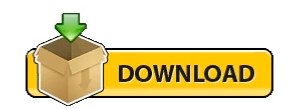
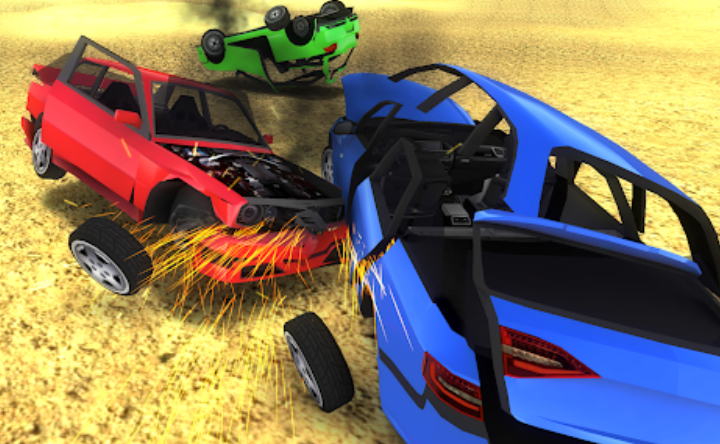
In particular we study recent nonlinear and sparse grid approaches, respectively. We combine clustering and nonlinear dimensionality reduction to show that the method is able to automatically detect parameter dependent structure instabilities or reveal bifurcations in the time-dependent behavior of beams. Here, we propose using methods from machine learning to semi-automatically analyze the arising finite element data and thereby significantly assist in the overall engineering process.
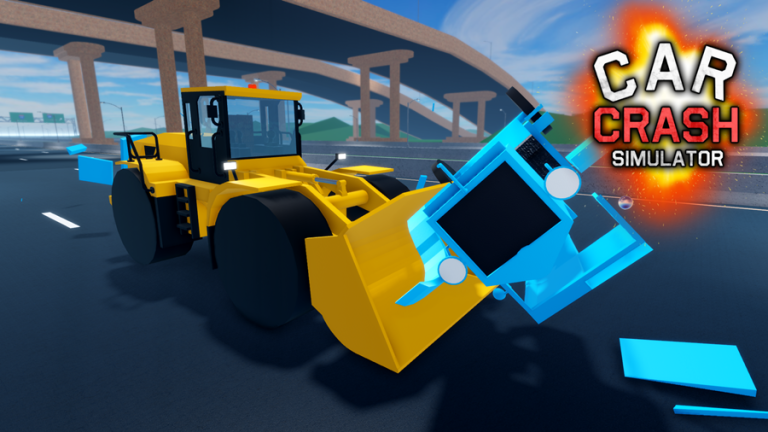
Car engineers spend a considerable amount of their time analyzing these influences by inspecting the arising simulations one at a time. For example, it is used to explore the influence of design parameters on the weight, costs or functional properties of new car models. Nowadays, product development in the car industry heavily relies on numerical simulations.
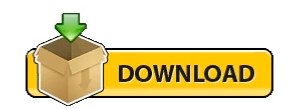